About Me
I am a senior scientist in the SciML team based at Rutherford Appleton Laboratory. SciML is a team in the Scientific Computing Division and we work with the large STFC facilities (Diamond, ISIS Neutron and Muon Source and Central Laser Facility for example) to use machine learning to push the boundaries of fundamental science.
My interests lie in developing machine learning approaches for materials science. I have experience leading projects to apply deep neural networks as well as classical machine learning (eg support vector machines) as well as atomistic simulation (eg DFT) to projects in materials characterisation and design. I have more than 10 years experience collaborating with experimental researchers to use computational tools for accelerated and advanced analysis of results.
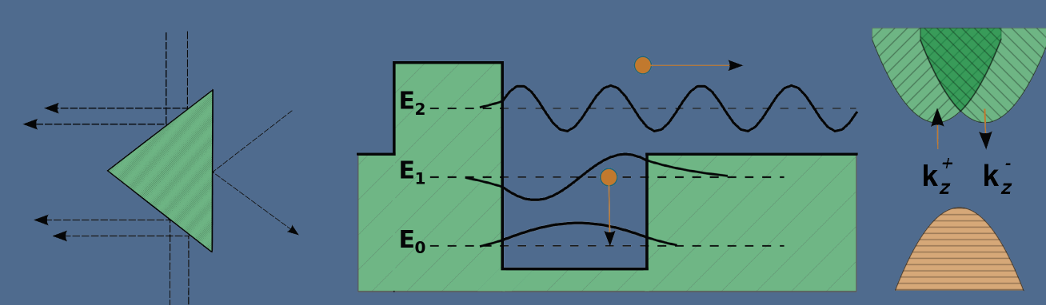
Projects
Machine Learning for Automated Experiments
With ISIS neutron and muon source are developing interpretable, calibrated machine learning techniques for the analysis of inelastic neutron scattering data. This work was recently highlighted by the British Computing Society here Also see my AI3SD webinar presentation here In work funded by Innovate UK, with Finden Ltd. we have been developing deep learning methods to reconstruct 3D maps of XRD patterns, allowing analysis of complex materials structures over unprecedented length scales and with unprecedented time resolution - opening up the potential for in situ observation of complex chemical phenomena in 3D. Collaborator Prof. Andy Beale presents the power of XRDCT here I also have projects with ePSIC and also Johnson Matthey we are building machine learning tools to enhance, accelerate and automate the operation of some of the world's most powerful electron microscopes.Machine Learning for Accelerated Materials Design
Developing descriptors, and tools for machine learning the properties of materials, from crystal structure prediction to property estimation. Unlock GNN allows use of modern graph neural networks to make use of knowledge about structure in the estimation of materials properties. When applied with SMACT this allows the construction of high-throughput design workflows for functional materials discovery.Software
During the past few years I have been leading development of and applying tools for materials science. These tools are available under open source licenses on my GitHub page.SMACT
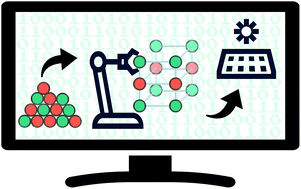
Superres-tomo

Macrodensity
